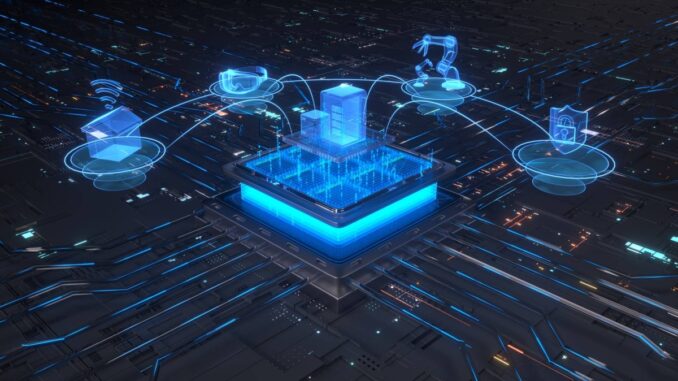
IDOESN’T take a genius to realize that artificial intelligence (AI) is transforming industries at an unprecedented rate.
According to IDC, global spending on AI is expected to reach $632 billion by 2028, with generative AI (GenAI) growing at a remarkable annual rate of 59.2 percent.
Yet, as AI capabilities surge, the infrastructure needed to support them is straining, impacting how quickly organizations can benefit from AI.
North American and Asian companies are champing at the bit for AI, with 76 percent of North American companies and 70 percent of Asian companies already starting their AI transformations. However, to maintain their edge, leaders must actively pursue transformation, said McKinsey, a business consultancy firm. Less than 10 percent of Asian organizations have found a way to drive value from multiple gen AI use cases. Those that do are likely to have a competitive advantage.
In the Philippines, companies already recognize the value of AI transformation. According to auditing and consultancy company, Deloitte, two-thirds (62 percent) of business leaders are excited about using AI, and more than three-quarters (79 percent) anticipate that GenAI will drive significant organizational transformation in less than three years.
Similarly, a report from PWC, another business consultancy firm, revealed that 78 percent of Philippine CEOs believe AI will improve the quality of their products and services, while 77 percent believe AI will increase the competitive intensity in their industries. However, the majority have yet to implement AI in the workplace (61 percent) and change their strategies (65 percent).
While GenAI drives much growth, it requires immense computing power, vast data storage, and advanced algorithms. Traditional infrastructures won’t be enough to support these demands, which may result in massive energy consumption, high costs, sustainability concerns, and even an impact on overall performance. Business infrastructure transformations are needed to ensure that any investments in AI are maximized.
Spending on AI infrastructure, including hardware such as servers and cloud infrastructure to support AI applications, is substantial but growing slower than GenAI adoption. According to IDC research, AI infrastructure will see a 14.7 percent compound annual growth rate (CAGR) through 2028, reflecting earlier investments by cloud service providers. AI hardware and infrastructure-as-a-service (IaaS) represent about 24 percent of overall AI spending, underlining its importance in unlocking AI capabilities. So, while GenAI is attracting increasing attention, AI infrastructure spending remains critical for supporting broader AI growth and applications.
For businesses eager to implement AI-driven solutions, investing in a robust, scalable and secure cloud infrastructure is now critical for success. But what does that AI infrastructure look like? What does AI explicitly need, and how can businesses transform accordingly?
Security, compliance capabilities
AI models process vast amounts of data. Data security and compliance with regulatory standards are essential for businesses deploying AI solutions. Secure infrastructure that includes encryption, robust access controls, and compliance with global data protection regulations (such as GDPR) will be needed to safeguard the models and the data they process.
In this regard, AI infrastructure must be designed for performance, scalability and security. It should be a standard consideration as failing to secure AI applications or the infrastructure supporting them can result in data breaches, regulatory fines, and loss of customer trust. Once trust has gone, it is almost impossible to regain.
Foundation for AI transformation
To meet AI’s growing demands, businesses must adopt cloud-native infrastructure, which includes powerful computing, high-performance network and storage, and container and data management systems. Cloud-native infrastructure provides the flexibility and scalability to support AI’s increasing computational and storage requirements. Traditional infrastructures struggle to manage modern AI applications’ massive data flows and high-performance needs.
Cloud-native architecture, however, allows businesses to rapidly scale their infrastructure to accommodate fluctuating demands, ensuring that they have the computing power necessary for GenAI models and other data-heavy AI processes.
Cloud-native environments support the compute-heavy operations required by AI and provide essential agility, which allows businesses to deploy, manage and update AI applications more efficiently. Importantly, cloud-native platforms are designed to seamlessly integrate with AI development workflows, which means companies can innovate faster without being held back by infrastructural limitations.
Scalable, reliable, cost-efficient infrastructure
As AI use cases multiply, the need for scalable and cost-efficient cloud infrastructure for data management and analytics becomes increasingly critical. Scalable infrastructure-as-a-service (IaaS) and platform-as-a-service (PaaS) offerings guarantee that data can be stored, processed and accessed seamlessly, enabling faster and more accurate model training.
Efficient data pipelines, robust storage solutions, and streamlined retrieval systems are crucial for managing these large volumes of data before they can be used for model training. An innovative infrastructure allows customization and fine-tuning of models for specific use cases, improving the quality and relevance of AI applications and simplifying AI model development.
AI applications must be built on reliable infrastructure to provide a consistent and trustworthy user experience. Downtime and crashes can erode user trust and disrupt operations. A solid infrastructure minimizes the risk of disruptions by ensuring that resources are always available, thus maintaining high availability and uptime.
Efficient AI infrastructure not only supports performance but also helps manage costs. Businesses can avoid overspending on cloud or hardware resources by optimizing computing resources through distributed systems, containerization and serverless architectures. This cost efficiency is vital for scaling GenAI applications without breaking the budget.
Energy efficiency and sustainability
As AI workloads increase, so do energy consumption and costs. AI models, particularly GenAI, are power-hungry, which has led to concerns about the environmental impact of AI growth. Businesses increasingly know the need for energy-efficient infrastructure to support their AI initiatives without significantly raising their carbon footprints. Green datacenters, renewable energy sources, and energy-efficient hardware are becoming essential components of AI infrastructure strategies.
By optimizing power consumption and investing in sustainable practices, businesses can reduce operational costs while meeting their sustainability goals. As AI adoption accelerates globally, focusing on energy-efficient infrastructure will become a key differentiator for companies looking to align innovation with corporate social responsibility and a need to manage costs more closely.
So, as AI continues to evolve, businesses must address current infrastructure challenges and anticipate future shifts in the AI landscape. These shifts should include security, regulatory compliance, and technical and sustainable needs. The convergence of real-time decision-making, augmented working environments, and the rising demand for sustainability means businesses must be proactive in their infrastructure strategies.
The risk of falling behind is real, but so is the opportunity to lead in this transformative era of AI. The question is no longer whether to invest in cloud infrastructure modernization but how quickly organizations can leap to stay competitive.
Allen Guo is the general manager for the Philippines at Alibaba Cloud Intelligence, a global leader in cloud computing and artificial intelligence, providing services to enterprises, developers and government organizations.
Be the first to comment